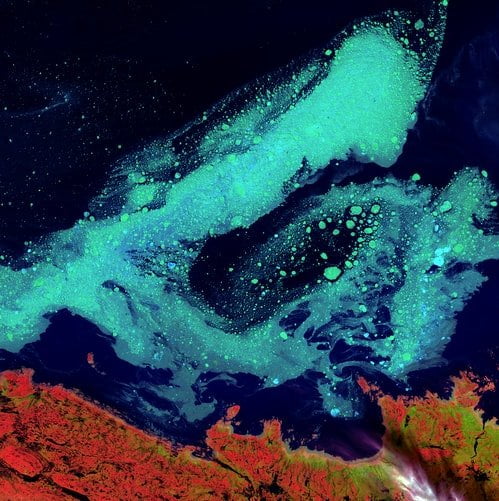
AI, Big Data, and the Environment – Part 1: Introduction
by Puria Radmard
The massive investments made [2], and the infamous Google purchase of DeepMind [3] in the mid 2010s made the use of data mining (a.k.a. artificial intelligence, or AI) in research and industry boom in the second half of the decade. Many non-profits and development hubs, a lot of them called ‘AI for Good’ – the summit, the UK organisation, and the US foundation – have been looking to source this interest in data science and direct it towards sustainability goals.
Of the three types of the UN’s Sustainable Development Goals (SDGs), environmental, economic, and social, the use of AI is predicted to aid environmental goals the most, and inhibit them the least [1]. This is in contrast, for example, to SDGs 4 and 10 (Quality Education and Reduced Inequalities) which see the highest inhibition from the rise of AI.
AIs aid to environmental action is mainly in decision making. While it can’t directly reduce emissions like renewable energy can, or sequester carbon itself, it can help make the best, most informed decisions in where to apply these, and the science underlying them.
Satellite imagery is a goldmine for AI applications. Computer vision, the branch of machine learning dealing with imagery and general spatial patterns, can help us survey land and understand geographical trends at a fraction of the cost or time it would usually take us. This can help with understanding consumption patterns [4], or combine this with data for wind speed, temperature, and other factors, and you can predict the shape of arctic ice with about 90% accuracy. [5]
Alongside research, AI’s private sector has found success in environmental services. In London, greyparrot.ai uses computer vision to automatically sort waste and recycling. Since this helps avoid the social cost of incorrect recycling [6], this technology can take out a big hurdle to being a sustainable individual. On an organisational level, cervest, also based in London, help climate risk and weather modelling. Harnessing data mining can help show us how and where to adapt, and what to expect (we’ll be talking about this more soon).
There are also more options to easily apply 3rd party AI technologies to research projects and company ventures, and more and more environmentalists are feeling the advantages of this new technology. See some of the groundbreaking applications in the last article in this series. [Part 4]
But there is a cost. Next time, we’ll discuss the environmental strain of the datasphere and the internet, including training machine learning models. [Part 2][1] https://www.nature.com/articles/s41467-019-14108-y
[2] https://www.edge.org/response-detail/26220
[3] https://www.theguardian.com/technology/2014/jan/27/google-acquires-uk-artificial-intelligence-startup-deepmind
[4] https://science.sciencemag.org/content/353/6301/790
[5] https://presentations.copernicus.org/EGU2020/EGU2020-15481_presentation.pdf
[6] https://www.bbc.co.uk/news/uk-wales-34920730